The workshop will take place tentatively on August 04, 2022, on Zoom and Youtube Livestreaming
About The Data Science Summer School
The Data Science Summer School is a series of theoretical and practical workshops on the exciting methods and technologies currently employed by industry, government, and civil society to address the world's most complex problems today. It is organized by the Hertie School Data Science Lab with funding and support from the Hertie School.
Workshop Details
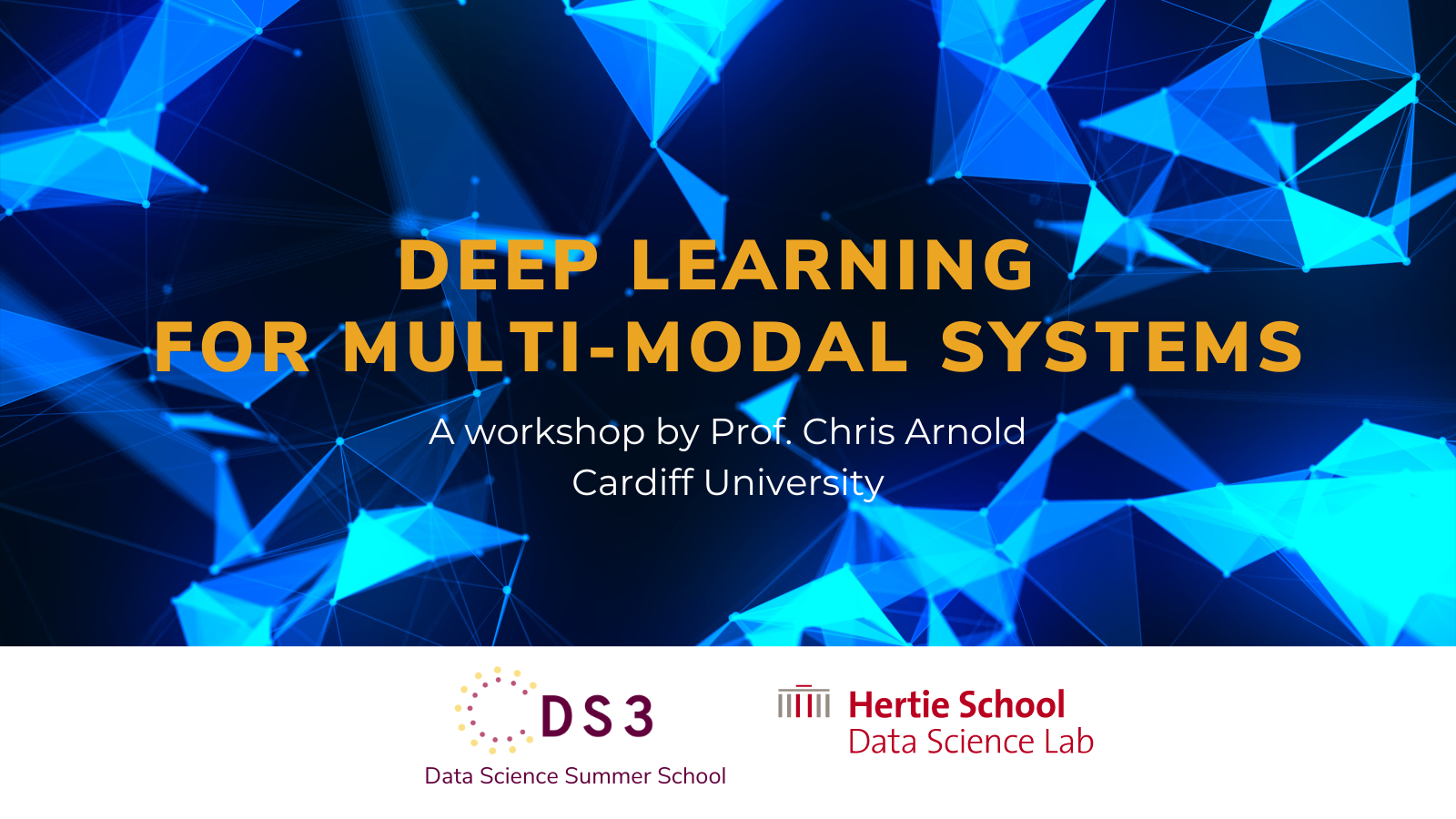
Humans experience the world often with more than just one modality: for example, when we watch a speaker we see them, we listen to their words, and we also get information from their tone. As social scientists who seek to explain the social world around us, we would often like to make use of all this rich information and not "just" focus on one modality alone for our studies. Over the past decade, computer science has made important advances in multimodal machine learning. There is now a powerful toolkit that helps analyze multiple modalities at once.
This course offers an introduction into the basics of multimodal machine learning and discusses multimodal representations, their alignment, their fusion and how to make neural networks co-learn from multiple modalities. During the module you will understand the relevant core concepts of multimodal learning. Using key neural network architectures, you will be able to train joined representations and apply them to supervised classification tasks.
As a student, it would be good to already have a solid understanding of neural networks and also how to use them for analyzing language and images. You ideally feel comfortable working in R and/or Python.
Core Readings
Baltrušaitis, Tadas, Chaitanya Ahuja, and Louis-Philippe Morency. "Multimodal machine learning: A survey and taxonomy." IEEE transactions on pattern analysis and machine intelligence 41.2 (2018): 423-443.
Bengio, Yoshua, Aaron Courville, and Pascal Vincent. "Representation learning: A review and new perspectives." IEEE transactions on pattern analysis and machine intelligence 35.8 (2013): 1798-1828.
Ian Goodfellow, Yoshua Bengio and Aaron Courville (2016), Deep Learning, MIT Press. (for free: http://www.deeplearningbook.org)
Content Licensing
All workshop materials and recording are under Creative Commons Attribution-NonCommercial-ShareAlike 2.0 license. You are free to share — copy and redistribute the material in any medium or format, and adapt — remix, transform, and build upon the material. However, you must give appropriate credit, provide a link to the license, and indicate if changes were made. You may do so in any reasonable manner, but not in any way that suggests the licensor endorses you or your use. You may not use the material for commercial purposes. If you remix, transform, or build upon the material, you must distribute your contributions under the same license as the original.
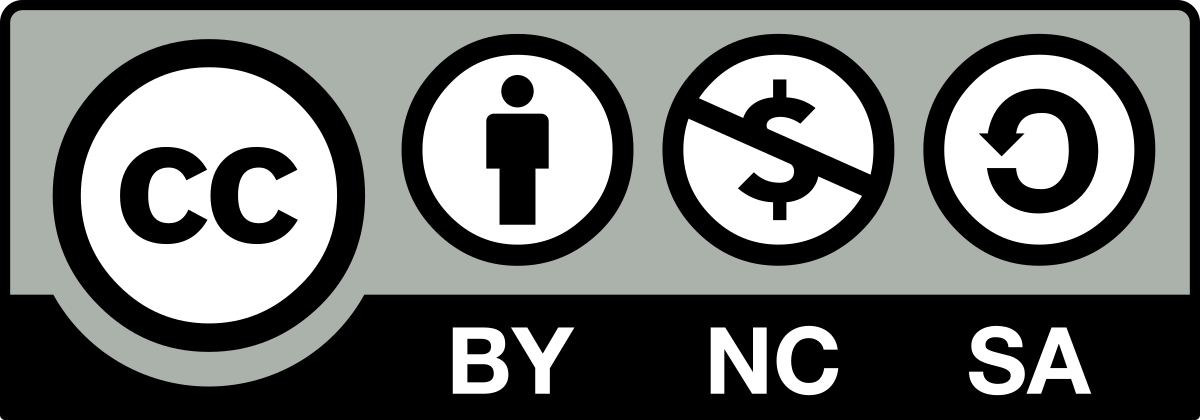
Workshop Materials
Instructor
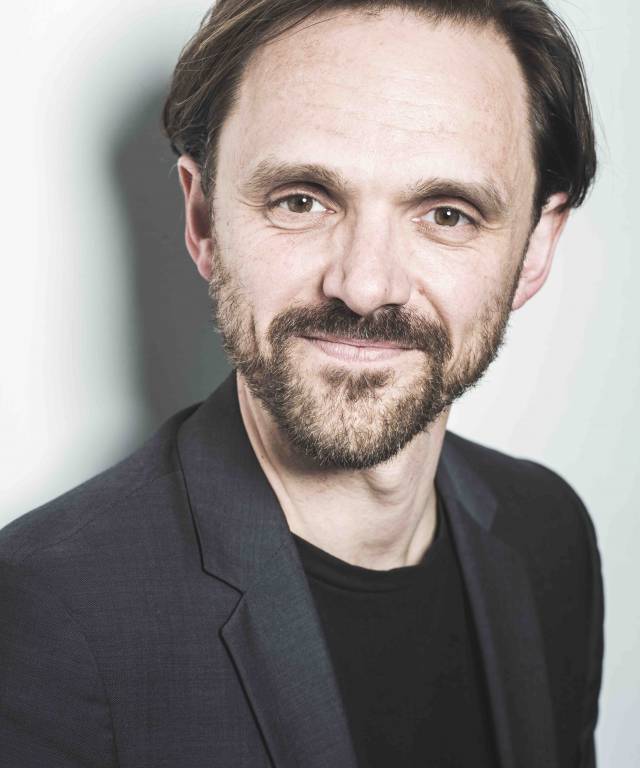
Prof. Christian Arnold
Prof. Christian Arnold is a Senior Lecturer at Cardiff University. Using data driven methods from statistics and machine learning, his work lies at the intersection between social science and computer science. His substantive research focuses on institutions in governance. What drives and determines the rules of political decision making? Prior to joining Cardiff, he had a position at Oxford University and worked as a Data Scientist in industry. He is a member of the academic advisory board for the Government Statistical Service at the Office for National Statistics. His focus there is on all matters related synthetic data.
Schedule (Central European Summer Time - CEST)
Session Starts
Deep Learning for Multi-Model Systems (Part I)
Short Break
Session Continues
Deep Learning for Multi-Model Systems (Part II)